People participating in augmented and virtual realities are sharing significantly more information than previously understood through their motion data, two new UC Berkeley-led studies show.
Users can be identified using just minutes of their head and hand movements, researchers found. Movement data, which is collected and shared with companies and other players to fuel these worlds, can be used to infer dozens of details from age to disability status.
“Users are revealing way more information than they think. They're revealing it, not just to the device or application, but to all the other users. And there's very little that they can do to prevent that,” said Vivek Nair, the studies’ lead author and a Ph.D. student in Berkeley’s Department of Electrical Engineering and Computer Sciences. “That makes it a particularly salient threat.”
These privacy and security risks are currently most relevant to gamers, the most common consumers of immersive physical and virtual computer-generated environments. People bought almost 10 million virtual reality headsets in 2022, and these risks could become even more pervasive soon. Last week Apple announced its own mixed reality headset, which will compete with Meta to make virtual reality tools interesting and accessible to a mainstream audience.
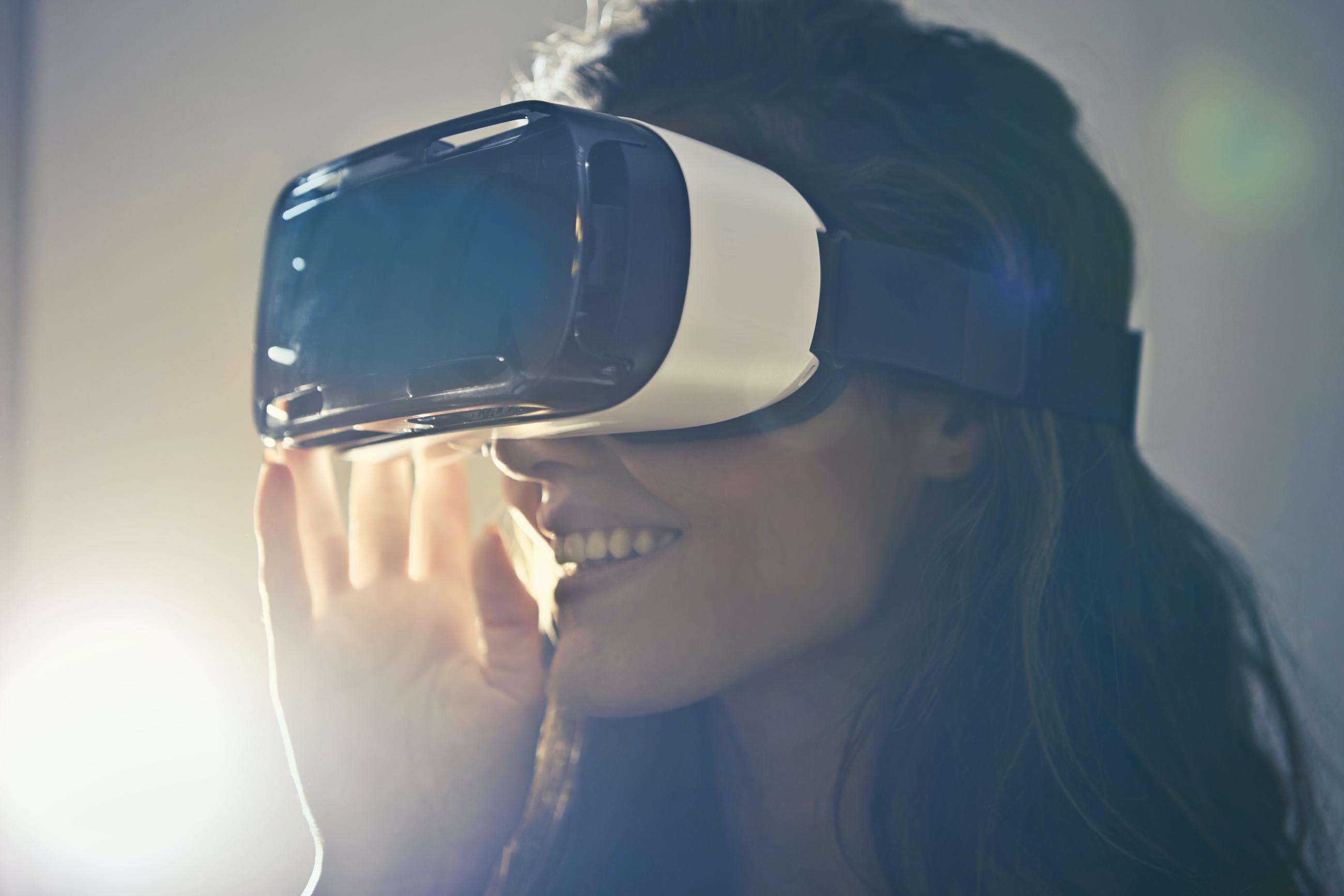
Both studies were completed through Berkeley’s Center for Responsible, Decentralized Intelligence, a multi-disciplinary initiative aimed at advancing the science, technology and education of decentralization and empowering a responsible digital economy. This work is part of the center’s Metaverse security and privacy research effort.
The paper, “Unique Identification of 50,000+ Virtual Reality Users from Head & Hand Motion Data,” is based on a dataset more than 100 times larger than those in past studies. Using open source data from more than 50,000 Beat Saber virtual reality gamers, it demonstrates that body movements are as singular and reliable an identifier as fingerprints.
The researchers trained their classification model on an individual player’s motion data for five minutes each. After that, the model could identify that user in just 10 and 100 seconds with 73 and 94 percent accuracy, respectively.
Authors include Berkeley’s Nair, Wenbo Guo, Rui Wang, James F. O’Brien and Dawn Song, as well as Unanimous AI’s Louis Rosenberg and Justus Mattern as a remote contributor. The paper has been peer reviewed and accepted for publication at the USENIX Security Symposium, a top conference for computer security and privacy research, that starts August 9.
A second paper, “Exploring the Unprecedented Privacy Risks of the Metaverse,” focuses on 50 participants in a lab setting. The academics created an adversarial virtual reality game aimed at collecting as much data as possible from the gamers in 10 to 20 minutes.
The team found they could accurately identify or infer more than 25 characteristics, including their location, age and height. Previous studies have found that almost all Americans can be correctly identified in any dataset using just 15 attributes. Due to ethical considerations, the Berkeley study didn’t attempt to ascertain more personal indicators like sexual or political preference. However, researchers believe these kinds of inferences may also be possible.
The study has been peer reviewed and will be published in July during the Privacy Enhancing Technologies Symposium. Nair and Song co-authored the study with Technical University of Munich’s Gonzalo Munilla-Garrido.
Nair intends to research defensive technologies to protect users’ privacy next. Without protections, bad actors could harness these worlds to, for example, steal identities or expose information that affects people’s employment opportunities or insurance costs, he said.
“We've done an extensive job of proving that there is a privacy risk here and that it is a different kind of privacy risk than what we have seen on the web,” Nair said. “These kinds of approaches for how to either transform the data or control who has access to it, that's going to be our main focus moving forward."