This article is cross-posted from
August 2 | UC Berkeley's College of Engineering
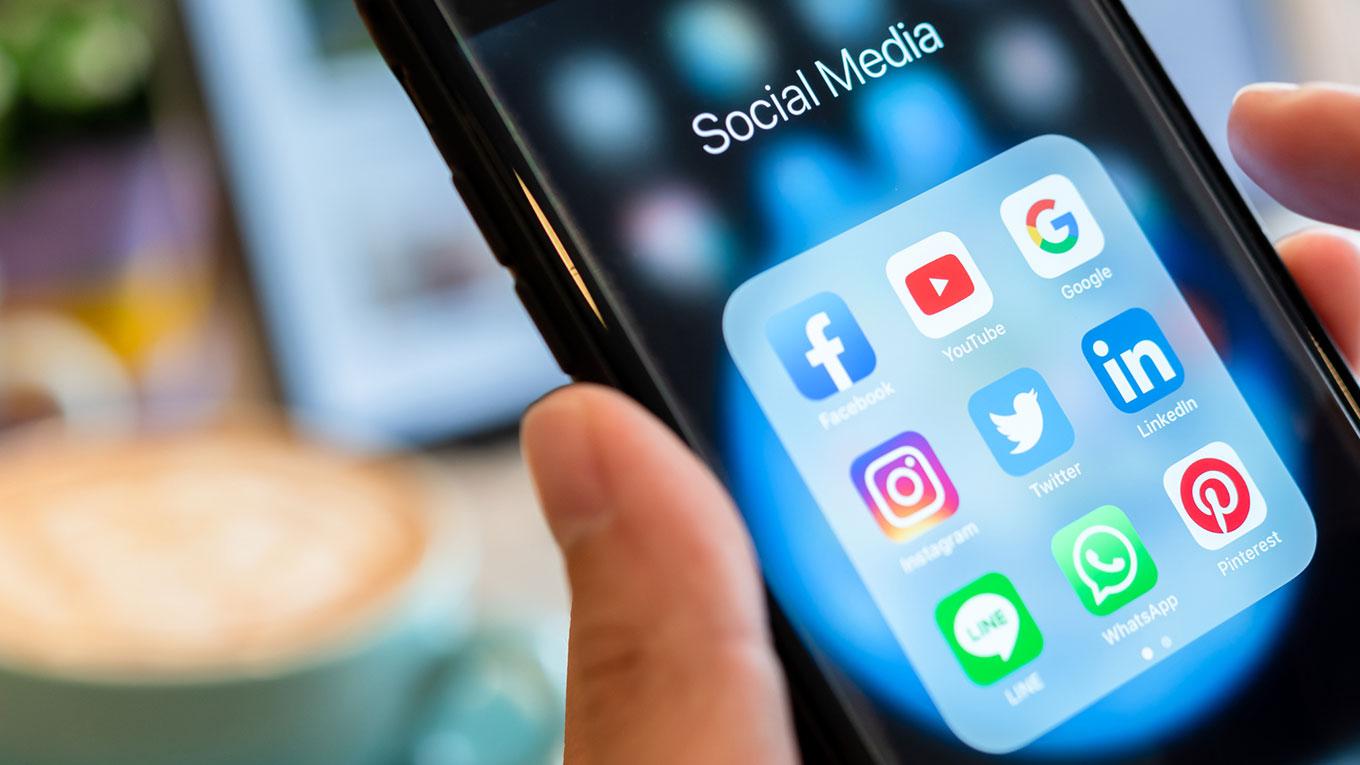
Scrolling through content selected “just for you” on social media feeds seems like a harmless pastime. But new research shows that some algorithms go from recommending content that matches our preferences to recommending content that shapes our preferences in potentially harmful ways.
In a study presented at the 2022 International Conference on Machine Learning, a UC Berkeley-led research team revealed that certain recommender systems try to manipulate user preferences, beliefs, mood and psychological state. In response, the researchers proposed a way for companies to choose algorithms that more closely follow a user’s natural preference evolution.
The study was conducted at the laboratory of Anca Dragan, associate professor of electrical engineering and computer sciences, with Micah Carroll, a Ph.D. student at the Berkeley Artificial Intelligence Research (BAIR) Lab; Stuart Russell, professor of electrical engineering and computer sciences; and Dylan Hadfield-Menell, now an assistant professor at MIT.
Recommender systems are algorithms that suggest content, such as posts, videos and products. They determine what we see on social media, what videos are recommended to us on YouTube and which ads should be shown to us on the internet. In this study, Dragan and her team focused on reinforcement learning (RL)-based recommender systems.
“These algorithms don’t just show us what we want to see right now, but optimize for our long-term engagement,” said Dragan. “The algorithm will show us content now that leads to the kind of preference changes that later can turn into even more engagement — whether or not that is aligned with what we would want.”
One way these manipulation incentives might manifest in theory is by driving certain users to embrace extreme positions, such as conspiracy theories. Imagine a person combing through political content on YouTube. Several clicks in, the content begins to shift, and the platform starts recommending content skewed toward political conspiracy theories. And as the user continues to click and watch videos, even out of curiosity, more of this type of content appears in order to keep the user engaged. Since it’s easier to keep people who like conspiracy theories engaged on the platform, there is the incentive to get users who would not normally watch that type of content to start appreciating it.
“Instead of making these algorithms dumber and hoping they won’t lead to undesired preference changes, we explored whether we could anticipate and even actively optimize against manipulation,” said Dragan. “To do this, though, you need a predictive model that tells you what influence a hypothetical new algorithm will have on real people and a way to evaluate whether that is manipulative or not.”
With these challenges in mind, the researchers set out to estimate how the preferences of users would be changed by a given recommendation algorithm before the algorithm is deployed. They also wanted to determine which preference shifts were likely the result of manipulation.
Their findings showed that using RL recommenders could lead to manipulative behavior toward users. “In our simulated experiments, the algorithm influenced users in ways that make their preferences more predictable to the system,” said Carroll. “The recommender can then methodically satisfy them — accumulating higher and more steady engagement levels.”
Seeking ways to develop recommenders that don’t exhibit a manipulation problem, the team attempted to model natural preference shifts, which are shifts that occur in the absence of a recommender. These natural shifts proved to be very different from preference shifts induced by the RL recommenders.
“By training recommenders to not deviate too much from natural shifts, while still optimizing key metrics like engagement, the system can learn that it should not dramatically alter users’ preferences,” said Carroll.
This study is particularly timely because RL recommenders are starting to be used on many popular platforms, including YouTube. And according to the researchers, the risks associated with using this technology warrant further investigation.
“While we focus on preferences, the risks extend well beyond that,” said Carroll. “The incentives that RL systems have for manipulating people may also include manipulating beliefs about the world, moods and psychological states more generally — potentially anything that can lead to high engagements on the platform.”
In the study, Dragan and her team proposed a framework for monitoring such risks. It could potentially be used by companies or auditors to assess whether current recommendation algorithms are already engaging in manipulative behaviors toward users; detect when they do so; and create new algorithms to avoid unintended manipulative or undesirable effects on preferences.
“We hope our research will be the foundation for additional work monitoring recommender system algorithms and their effects on users,” said Carroll.