As an undergraduate mathematics student 14 years ago, Jacob Steinhardt began to consider a future where artificial intelligence would automate most cognitive work, which he thought could have an even bigger impact than the Industrial Revolution.
“The future of AI seems pretty uncertain,” he remembers contemplating, “maybe by applying my math and computer science background, I could help things go well.”
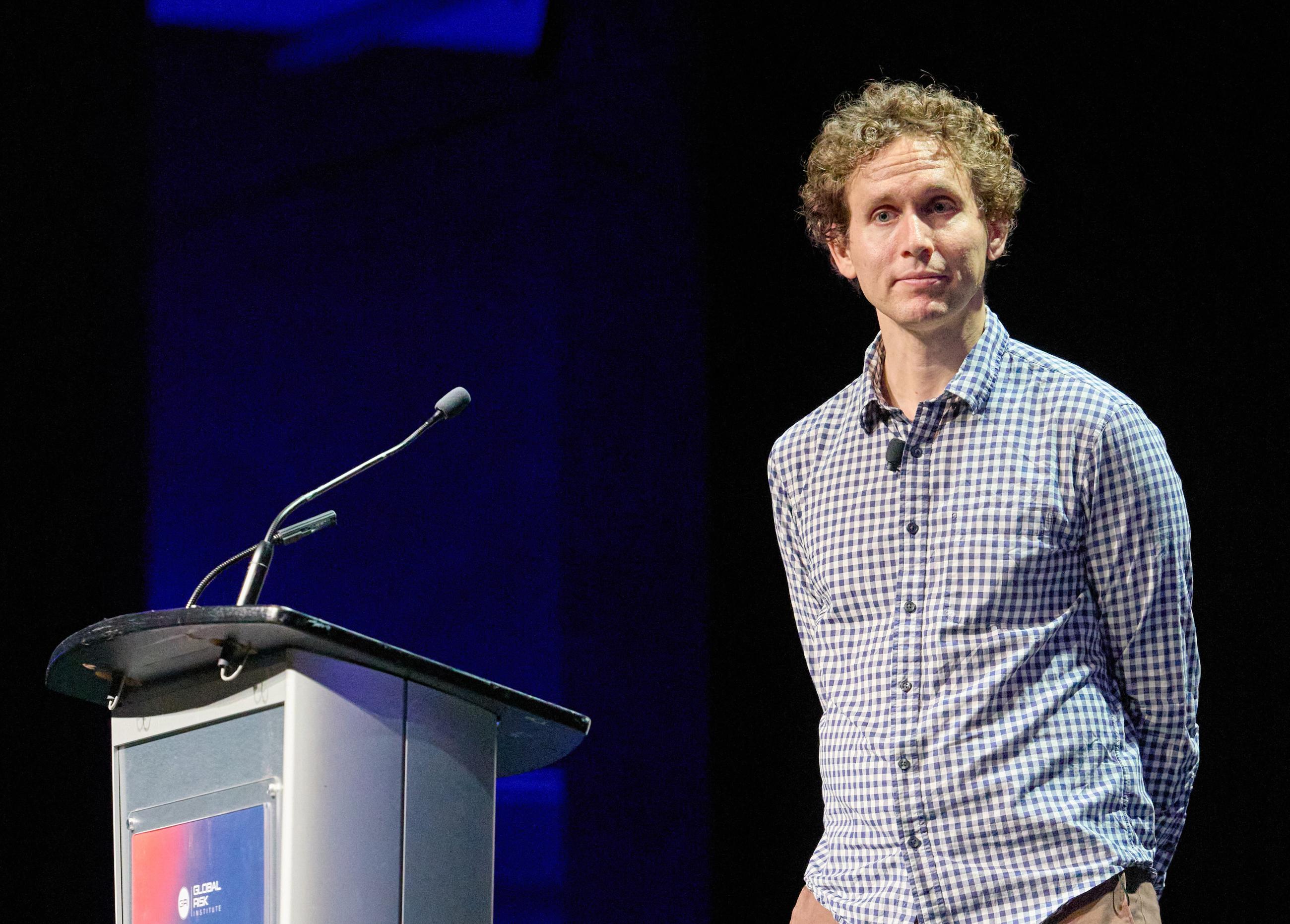
Today, Steinhardt is an assistant professor of electrical engineering and computer sciences and statistics at UC Berkeley and the co-founder of Transluce. He still considers himself a “worried optimist” regarding AI’s potential impact on society. But to direct its impacts in an optimistic direction, he works at the intersection of AI safety and public oversight, developing the technical tools needed to understand and govern AI systems.
“I focus on what we need to do to help steer that impact for the good of society and humanity,” Steinhardt said.
Aligning AI systems with human needs
Part of the challenge of aligning AI systems with human needs is that – unlike conventional software programs built to precise specifications – modern AI systems develop more organically through training on massive datasets. Steinhardt compares them to pets since their behavior can be shaped but not directly programmed, making them harder to predict and control. “You can't easily predict what your dog is going to do even though you've trained it,” he said. “AI systems have the same problem.”
This unpredictability, combined with their growing capabilities, could open doors for humans to lose control over AI systems or misuse them in intentionally harmful ways. Steinhardt suggested that in the future we will also likely have hundreds of millions of AI systems interacting with each other every day, so problems in individual AI systems could have ripple effects. “That's just messy, in the same way that human society is messy,” he said.
In his research, Steinhardt aims to make AI systems less opaque and more reliable. On the technical side, he's developing automated testing systems to evaluate AI models before deployment, like putting them through every possible scenario to discover problems in advance of real-world use. In addition, he's working on analyzing AI systems' processes to better understand their decision-making.
He’s also working to build mutually beneficial partnerships between developers of proprietary AI models and the open ecosystem. “There’s so much we can learn by studying the quality of these systems in the open,” Steinhardt said. “The public deserves access to that information, and it will also help companies produce more reliable systems overall.”
Open-source tools for auditing AI systems
Toward that vision, Steinhardt took a sabbatical from Berkeley in July 2023 and launched Transluce with co-founder Sarah Schwettmann. The startup aims to create open-source tools for auditing AI systems. The group’s goal is to independently and openly assess model safety instead of leaving evaluation behind closed doors.
“I just felt this very pressing need, and felt strongly called to do it,” Steinhardt said.
The company is developing ways to analyze terabytes of data to reveal how new AI systems think and better understand their capabilities and risks. They're also working with government entities concerned with AI security to support oversight efforts and work toward public audits of openly available models.
For Steinhardt, it’s important that Transluce systems are openly available because it allows other people to run similar audits themselves and to check their work. “A really big part of what's important and what's in our DNA is this idea of public vetting,” he said.
Transluce’s next big step is to run a large-scale audit for publicly available models, such as DeepSeek and Llama. He plans to perform a public audit with colleagues as a demonstration of how each model thinks through inputs and how to address problems such as AI “hallucinations,” which are false or misleading responses produced by the algorithm.
The value of cross-disciplinary thinking
Although Steinhardt is excited about launching the start-up, he misses teaching cross-disciplinary courses like Stat 165, Berkeley’s popular course on forecasting that combines statistical methods with data science and computer science.
Thinking across disciplines is something that Steinhardt values, both in his work and spare time. He enjoys reading across a broad range of fields including economics, biology and political science. He’s found that having diverse interests helps inform his work.
"I feel like I've often read things out of pure intellectual interest and then later found it to be very useful for research," he said. Most recently, Steinhardt has become interested in the history of biology and the mistakes and successes that led to biological breakthroughs, which he likens to how AI works through problems. "To me, history is just like a lot of training data," he said.
Steinhardt’s experience leaves him with hope that creative interventions can create safer and more reliable AI. “I think civilization is pretty robust,” he said. “I feel optimistic that if we try everything we can, we will come up with good paradigms for handling powerful AI systems.”